Welcome to the MALEO group
The Machine Learning and Optimisation (MALEO) group, led by Prof. Dr. Heike Trautmann, was established in October 2023 at Paderborn University, Germany. Our theoretical and empirical research mainly focuses on (Trustworthy) Artificial Intelligence, Data Science, (Automated) Machine Learning, Automated Algorithm Selection and Configuration, Exploratory Landscape Analysis, Analysis of Randomised Search Heuristics, and (Multiobjective) Evolutionary Optimisation. In interdisciplinary collaboration, we are moreover addressing Computational Social Science topics such as Social Influence Analysis and Disinformation Campaign Detection in Open Online Media. We have diverse and extensive national and international collaborations with particularly strong links to the University of Twente, Enschede. At the European level, we strongly support the Confederation of Laboratories for Artificial Intelligence Research in Europe (CLAIRE) and the European Research Center for Information Systems (ERCIS).
We refer the reader to the official MALEO-group research-website for more information.
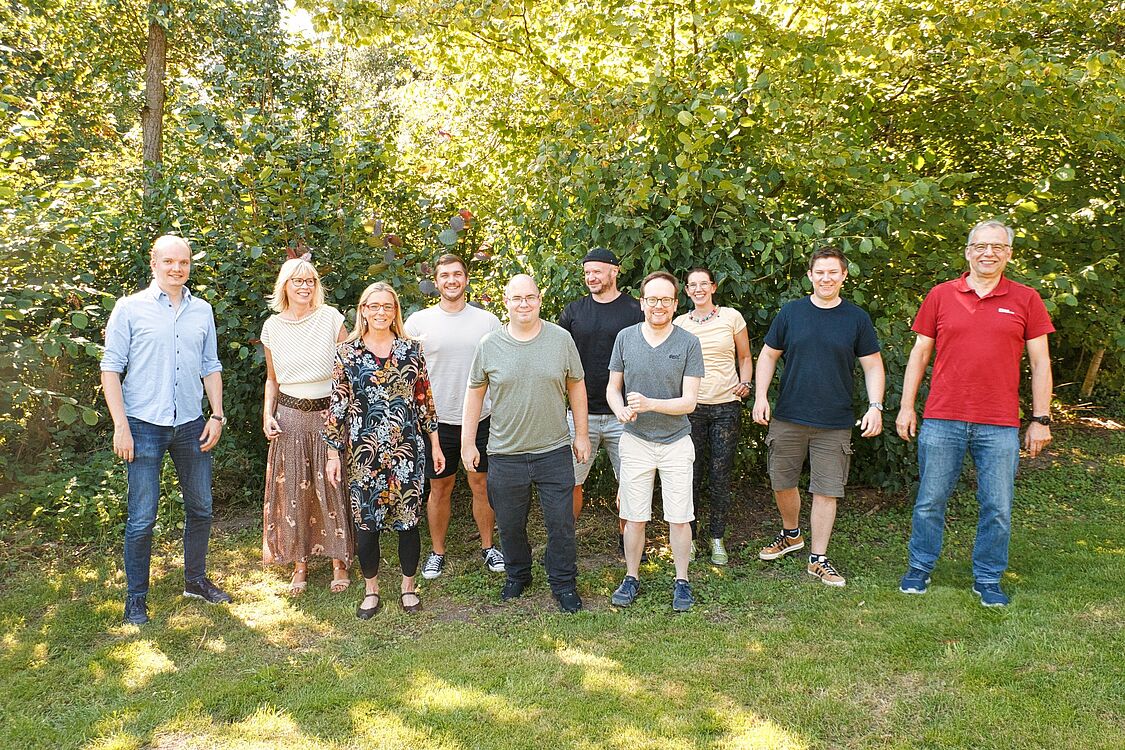
Recent and Past Teaching
- Lecture: Angewandte Statistik mit R
Lecturer: Prof. Dr. Heike Trautmann
The lecture provides an overview of descriptive statistics and exploratory data analysis as well as inductive statistics and the basics of probability theory. In addition to conveying an intuitive understanding of respective methods, a further focus is on applied data analysis making use of the statistical programming language R, which is introduced in the first block of the lecture as part of a programming course. No prior knowledge of R is required.
- Lecture: Multi-Objective Optimisation
Lecturer: Dr. Jakob Bossek
Optimization problems are ubiquitous, and we all (approximately) solve them in everyday life, such as when finding routes with Google Maps to quickly get from point A to point B or deciding on a checkout lane with the shortest waiting queue (shortest expected waiting time) at the supermarket. However, optimization problems are rarely single-criteria. Instead, they are typically multi-criteria in nature, with the individual objectives usually conflicting with each other. For example, in route planning, the distance traveled may be relevant (shorter is better), and fuel consumption may also be a consideration (lower is better). The shortest route may lead through the city center with many stop-and-go maneuvers at red lights, especially during peak hours. On the other hand, a longer route around the city may consume less fuel. Accordingly, the goal in multi-objective optimization is to find a set of optimal compromise solutions.
This course provides a comprehensive introduction to multi-objective optimization and the associated challenges. In addition to classical general approaches, exact methods for selected combinatorial optimization problems are presented, along with heuristic (nature-inspired) methods. The course also covers heuristic solution approaches for problems with more than three criteria (many-objective optimization).
- Seminar: Machine Learning Assisted Evolutionary Multi- and Many- Objective Optimization
Lecturer: Prof. Dr. Heike Trautmann
This seminar, partly based on an upcoming respective book by Saxena, Deb et al. (2024), focuses on machine learning (ML) assisted evolutionary multi- and many-objective optimization (EMO). EMO algorithms, namely EMOAs, iteratively evolve a set of solutions towards an optimal trade-off surface, i.e. Pareto Front. The availability of multiple solution sets over successive generations makes EMOAs amenable to application of ML for different pursuits, a large proportion of optimization problems in practice is multi-objective in nature.
We will cover the foundations of optimization, including problem and algorithm types and then focus on key studies on ML-based enhancements in the EMO domain, systematically addressing important aspects. Moreover, we will discuss Auto-ML based techniques such as Automated EMOA configuration. The seminar will have two phases, theoretical will be followed by experimental work in groups.
- Seminar: Data Stream Mining
Lecturer: Prof. Dr. Heike Trautmann
This seminar will focus on the characteristics of Data Streams and related Data Stream Mining algorithms. We will mainly concentrate on textual data in the social networks domain and discuss application areas such as Disinformation Campaign Detection or Synthetic Social Network Data Generation. The seminar will have two phases, theoretical will be followed by experimental work in groups.
- Proseminar: Evolutionary Computation
Lecturer: Dr. Jakob Bossek
The key idea of this seminar is to dive into diverse topics in the context of evolutionary computation. Evolutionary algorithms (EAs) are bio-inspired randomized search heuristics that mimic principles from natural evolution to “evolve” good solutions iteratively. Such algorithms particularly shine in the context of finding good solutions for black-box optimization problems (where knowledge about the function at hand is obtainable via queries only) or (multi-objective) NP-hard combinatorial optimisation problems.
-
Project Group: Next Gen User Interface for optimization software based on Generative AI (GenUIne)
Lecturer: Dr. Moritz Seiler, Prof. Dr. Heike Trautmann
Using generative AI we are going to re-invent the next gen User Interface for optimization software in close cooperation with the company Optano (https://optano.com).
Optimization software (e.g. https://optano.com/en/solutions/) has a wide range of use cases and features, it is hard to learn and complex to use, especially when users are no domain experts or rarely use such tools. For those users the power of optimization remains a locked secret.
This project will demonstrate how latest generative AI, e.g. LLMs, can support users to understand complex software products, get insight in their data and run complex tasks automatically and without deep knowledge of the tool. Users will interact with optimization software using their mother tongue. We will create a great experience for users. We leverage GenAI. We are GenUIne!
Planned Lectures
- Lecture: Programming (Bachelor, German, 4V + 2Ü)
Software development is a central area of work in computer science. Software developers must be able to analyze and model tasks, design software structures, and implement them in a programming language. This module imparts introductory and scientifically based knowledge and skills in programming. Together with the modules Programming 2, Programming Languages, Software Engineering, and the Software Engineering Internship, it establishes and practices the scientific foundations for the field of software development. This module aims to enable participants to apply a programming language relevant to software development (currently Python, to a lesser extent Java), to use basic concepts of object-oriented programming methodology, and to implement algorithms in programs.
- Lecture: Unsupervised Learning and Evolutionary Computation Using R (Master, English, 3V + 2Ü)
- Project Group: (Master, English) Next Gen User Interface for optimization software based on Generative AI (GenUIne)
Projects
Using rail mobility to contribute to highly automated, digitalised and sustainable mobility and to rethink interfaces with other modes of transport - this is the aim of the project "Automated rail transport as the basis for sustainable, networked mobility in rural areas (enableATO)", in which researchers from Paderborn University are involved. The project will be based at the RailCampus OWL in Minden. Minden is thus one of four locations within the DZM (German Centre for Future Mobility).
Over the next three years, a strong project consortium of universities, Fraunhofer institutes in the region and companies will advance technologies for automated, rail-based mobility concepts and research and identify interfaces between rail-based mobility and other modes of transport in rural areas. Driverless rail transport systems such as the MONOCAB or the road-rail vehicle will demonstrate automated rail transport in Minden and Extertal. The aim is to use them in regular test operations in the near future.
The funding of 12.5 million euros is being provided by the Federal Ministry for Digital and Transport Affairs (BMDV).
The aim of the "enableATO" project is to enable modern ideas for automated rail mobility and their investigation in new rail-based approaches in rural areas. The focus is on technologies related to automated driving such as perception by sensors, authorisation issues, intelligent maintenance and the demonstration of technologies, e.g. in the MONOCAB. At the same time, initial questions regarding user acceptance are being researched and addressed and the scientific dialogue strengthened.
The challenge of transport system transformation is to combine sustainability and efficiency. Automation, autonomous driving, intelligent traffic management, digital connectivity and networked mobility play a central role in this context. The RailCampus OWL as an innovation centre for this project provides space for new impulses and makes a decisive contribution to the research and development of new automated mobility approaches for sustainable rail mobility as a socially relevant factor.
Investigating rail-bound mobility at the RailCampus OWL
The RailCampus OWL pools and expands expertise and will create and utilise new things in this project. Various new mobility approaches are being researched and demonstrated there, such as automated transport with the MONOCAB developed by TH OWL and a two-way vehicle that was initially designed at Bielefeld University of Applied Sciences and Arts for logistics applications and can travel on both rail and road. These approaches aim to reactivate unused railway infrastructure and use it sustainably for more individualised mobility by rail, but require automation.
Strong partnership for sustainable mobility
The project consortium is made up of renowned partners. In addition to Paderborn University, the consortium includes Bielefeld University, Bielefeld University of Applied Sciences and Arts, Ostwestfalen-Lippe University of Applied Sciences and Arts, DB Systemtechnik GmbH, Wölfel Engineering GmbH + Co KG, HARTING Technology Group, Pilz GmbH & Co. KG and the Fraunhofer Institutes IEM (Paderborn) and IOSB-INA (Lemgo). Associated partners are WAGO and DB Cargo AG. Together, the partners are contributing extensive expertise and many years of experience to advance the vision of sustainable, networked and automated mobility in rural areas. The enableATO project will make an important contribution to this.
Algorithm-based approaches, such as machine learning, are becoming increasingly complex. This lack of transparency only makes it more difficult for human users to understand and accept the decisions proposed by artificial intelligence (AI). In response to these societal challenges, computer scientists have begun to develop self-explanatory algorithms that provide intelligent explanations (so-called “explainable artificial intelligence” – XAI). XAI programs, however, only interact with humans to a limited extent and do not take into account the given context or the types of information the humans being addressed need. This runs the risk of generating AI explanations that will not be understood by the human user.
Members of the Transregional Collaborative Research Centre “Constructing Explainability” (TRR 318) are challenging this view by instead conceiving of explanation as a process of co-construction: in this model, the person receiving the explanation takes an active role in the AI explanatory process and co-constructs both the goal and the process of explanation. In a collaborative, interdisciplinary approach, the mechanisms of explainability and explanations are being investigated by 21 project leads assisted by approximately 30 researchers from fields as diverse as linguistics, psychology, and media studies, to sociology, economics, and computer science.
The findings of the research efforts from TRR 318 will contribute to the development of:
- A multi-disciplinary understanding of the explanatory process linked to the process of understanding and the contextual factors influencing it.
- Computer models and complex artificial intelligence systems that can generate situation-specific and efficient explanations for their addressees.
- A theory of explanation as social practice that considers the expectations of the person being communicated with, as well as their role in the interaction.
These foundations for explainable and understandable artificial intelligence systems will enable greater active and critical participation in the digital world.
This COST Action aims at making randomised optimisation algorithms widely competitive in practice by identifying and reducing obstacles to their adoption at the scientific, technical, economic, and human levels. It focuses on meeting the needs of practitioners, from whose activities the economic value of optimisation solvers stems. These needs are taken as the driving force for new theoretical, methodological, and technical advances leading to the sustainable development of widely available software tools, training materials and programmes, and ultimately to more extensive acceptance and deployment of these methods.
Publications
2024
@article{Prager_Trautmann_2024, title={Exploratory Landscape Analysis for Mixed-Variable Problems}, DOI={10.1109/TEVC.2024.3399560}, journal={IEEE Transactions on Evolutionary Computation}, author={Prager, Raphael Patrick and Trautmann, Heike}, year={2024}, pages={1–1} }
@article{Rodriguez-Fernandez_Schäpermeier_Hernández_Kerschke_Trautmann_Schütze_2024, title={Finding ϵ-Locally Optimal Solutions for Multi-Objective Multimodal Optimization}, DOI={10.1109/TEVC.2024.3458855}, journal={IEEE Transactions on Evolutionary Computation}, author={Rodriguez-Fernandez, Angel E. and Schäpermeier, Lennart and Hernández, Carlos and Kerschke, Pascal and Trautmann, Heike and Schütze, Oliver}, year={2024}, pages={1–1} }
@inproceedings{Dietrich_Prager_Doerr_Trautmann_2024, place={Cham}, title={Hybridizing Target- and SHAP-encoded Features for Algorithm Selection in Mixed-variable Black-box Optimization}, booktitle={Parallel Problem Solving from Nature — PPSN XVIII}, publisher={Springer International Publishing}, author={Dietrich, K. and Prager, R. and Doerr, C. and Trautmann, Heike}, editor={Affenzeller, M and Winkler, S and Kononova, A and Trautmann, H and Tušar, T and Machado, P and Baeck, T}, year={2024}, pages={1–14} }
@inproceedings{Seiler_Skvorc_Cenikj_Doerr_Trautmann_2024, place={Cham}, title={Learned Features vs. Classical ELA on Affine BBOB Functions}, booktitle={Parallel Problem Solving from Nature — PPSN XVIII}, publisher={Springer International Publishing}, author={Seiler, Moritz and Skvorc, Urban and Cenikj, G and Doerr, C and Trautmann, Heike}, editor={Affenzeller, M and Winkler, S and Kononova, A and Trautmann, H and Tušar, T and Machado, P and Baeck, T}, year={2024}, pages={1–14} }
@inproceedings{Seiler_Rook_Heins_Preuß_Bossek_Trautmann_2024, title={Using Reinforcement Learning for Per-Instance Algorithm Configuration on the TSP}, DOI={10.1109/ssci52147.2023.10372008}, booktitle={2023 IEEE Symposium Series on Computational Intelligence (SSCI)}, publisher={IEEE}, author={Seiler, Moritz and Rook, Jeroen and Heins, Jonathan and Preuß, Oliver Ludger and Bossek, Jakob and Trautmann, Heike}, year={2024} }
@inproceedings{Rook_Hoos_Trautmann_2024, title={Multi-objective Ranking using Bootstrap Resampling}, DOI={10.1145/3638530.3654436}, booktitle={Proceedings of the Genetic and Evolutionary Computation Conference Companion, GECCO 2024, Melbourne, VIC, Australia, July 14-18, 2024}, publisher={ACM}, author={Rook, Jeroen and Hoos, Holger H. and Trautmann, Heike}, editor={Li, Xiaodong and Handl, Julia}, year={2024}, pages={155–158} }
@inbook{Preuß_Rook_Trautmann_2024, place={Cham}, title={On the Potential of Multi-objective Automated Algorithm Configuration on Multi-modal Multi-objective Optimisation Problems}, DOI={10.1007/978-3-031-56852-7_20}, booktitle={Applications of Evolutionary Computation}, publisher={Springer Nature Switzerland}, author={Preuß, Oliver Ludger and Rook, Jeroen and Trautmann, Heike}, year={2024} }
2023
@article{Prager_Trautmann_2023, title={Pflacco: Feature-Based Landscape Analysis of Continuous and Constrained Optimization Problems in Python}, DOI={10.1162/evco_a_00341}, journal={Evolutionary Computation}, author={Prager, Raphael Patrick and Trautmann, Heike}, year={2023}, pages={1–25} }
@article{Bossek_Sudholt_2023, title={Do Additional Target Points Speed Up Evolutionary Algorithms?}, DOI={10.1016/j.tcs.2023.113757}, journal={Theoretical Computer Science}, author={Bossek, Jakob and Sudholt, Dirk}, year={2023}, pages={113757} }
@article{Bossek_Grimme_2023, title={On Single-Objective Sub-Graph-Based Mutation for Solving the Bi-Objective Minimum Spanning Tree Problem}, DOI={10.1162/evco_a_00335}, journal={Evolutionary Computation}, author={Bossek, Jakob and Grimme, Christian}, year={2023}, pages={1–35} }
Show all publications
Contact
Marion Hucke
Machine Learning and Optimisation
Fürstenallee 11
33102 Paderborn
Office hours
Monday afternoon
Wednesday afternoon
Thursday morning